Experiment 11 - CloudEcho: Cloudified analysis of multi-sensorial data for optimised manufacturing
Experiment description
Hard metal industry manufactures high precision (order of μm), hard metal tools and parts for heavy duty and critical applications in fields such as mining, oil drilling, surgical instruments and industrial cutting tools. The raw material used is Tungsten Carbide (WC) given its superior mechanical and chemical properties over steel and aluminium alloys. In each production phase, the part is manually inspected for dimensional or structural defects (e.g. geometry deviation, crack and pores). Any of these, depending on the phase it occurred, leads to rejection of the part. CloudEcho aims to optimize this process by the cloudification and automation of inspection and defect prediction.
To this end, Deep Neural Networks (DNNs) will be deployed for analysing data from;
i) shop-floor sensors on processing machines measuring vibration, temperature and energy consumption,
ii) micro-profilometer scans, capturing the surface geometry of processed parts with high accuracy (below 5 micro-meters), and
iii) ultra sound scans, non-destructively penetrating the inner structure of processed parts, allowing the detection of internal defects and the inspection of non-accessible areas (e.g. cavities, small holes) that cannot be manually examined.
Technical impact
In a nutshell, the main question that CloudEcho addresses is whether a manufacturing process in the hard metal industry can advance from manual inspection and production planning to automated tools for the defect detection and prediction. From a scientific point of view, the novelty of CloudEcho lies on the deployment of deep learning techniques for the cloud analysis of multimodal sensory signals and particularly in the combination of ultrasound and microprofilometry scans for the identification of defects in the internal structure of hard metal parts that will drastically improve the robustness and reliability of produced items. The potential of the proposed technology will be verified early on the lifecycle of the experiment, after the first round of data collection completes and initial experiments are conducted on whether defects (even internal/hidden ones) can be identified.
The process that with CloudEcho the experiment wants to achieve is divided in three blocks:
- The first block will collect data from three sources: the micro-profilometry surface scans, ultra sound volume scans and the shop-floor sensor data.
- The second block will joint the multimodal analysis based on Deep Learning
- And the third block will show the visualization and early user notification
Economic impact
The economic impact of CloudEcho can be pinpointed to the following:
- Production time savings from preventing defects through prediction and not allowing defective parts to propagate in the manufacturing process. Depending on the stage of processing, the cost of the part is from about 50 to 75% of the total, so a loss of remaining value of 25 to 50% is expected.
- Reduced personnel costs, due to automated rather than manual defect inspection.. An estimated 3-5% of the total cost is due to manual inspection throughout the entire production line.
- Identified defects that couldn’t be manually detected. Here the lost cost of the part could be in the range of 50 to 100% the total value of the part.
- Energy savings stemming from fewer parts having to be reworked and from fewer scrap parts having to be dissolved. From 10 to 12% is the expected added value of energy to the cost of each part.
- Savings in raw material (WC powder) due to reduce rate of scrap parts. The cost is manifold and includes reprocessing of powder (milling, spray drying and green machining) and opportunity costs of having to reallocate resources to produce replacement parts.
Experiment Outcome
CloudEcho experiment created an automated quality inspection system based on deep neural networks for the hard metal industry. The system makes early detection of defects possible before a defect is replicated in several parts.
CloudEcho experiment further was able to identify the machine deterioration from the 1st defective part. This allows the maintenance of equipment and avoid producing more defective part and translates to don’t have to reproduce parts, sparing energy and raw materials.
- The developed models achieve accuracy higher than 75%.
- The microprofilometer model achieved general accuracy 77.78%.
- The ultrasound model achieved general accuracy 83.33%.
- The fusion model scored an accuracy of 88.24%.
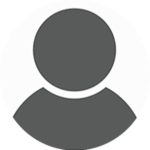
“CloudEcho optimizes the production system and decreases the reproduction of defects in an accurate and solid way.”
Athanasios Kotsiopoulos, Research Assistant at ITI, Greece.